AI-Generated Prior Art: Navigating the Future of Patent Examination
The unprecedented scale of content generated by AI presents an interesting challenge, in terms of prior art, to established patent law practice. With AI capable of autonomously producing technical content, future patent applications may face an unprecedented wave of AI-generated prior art disclosures that could be relevant in the assessment of novelty and nonobviousness (or inventive step). This article explores how AI-generated content may reshape how we consider prior art, and what might need to be done, as we navigate a new era of innovation influenced by machine-generated insights.
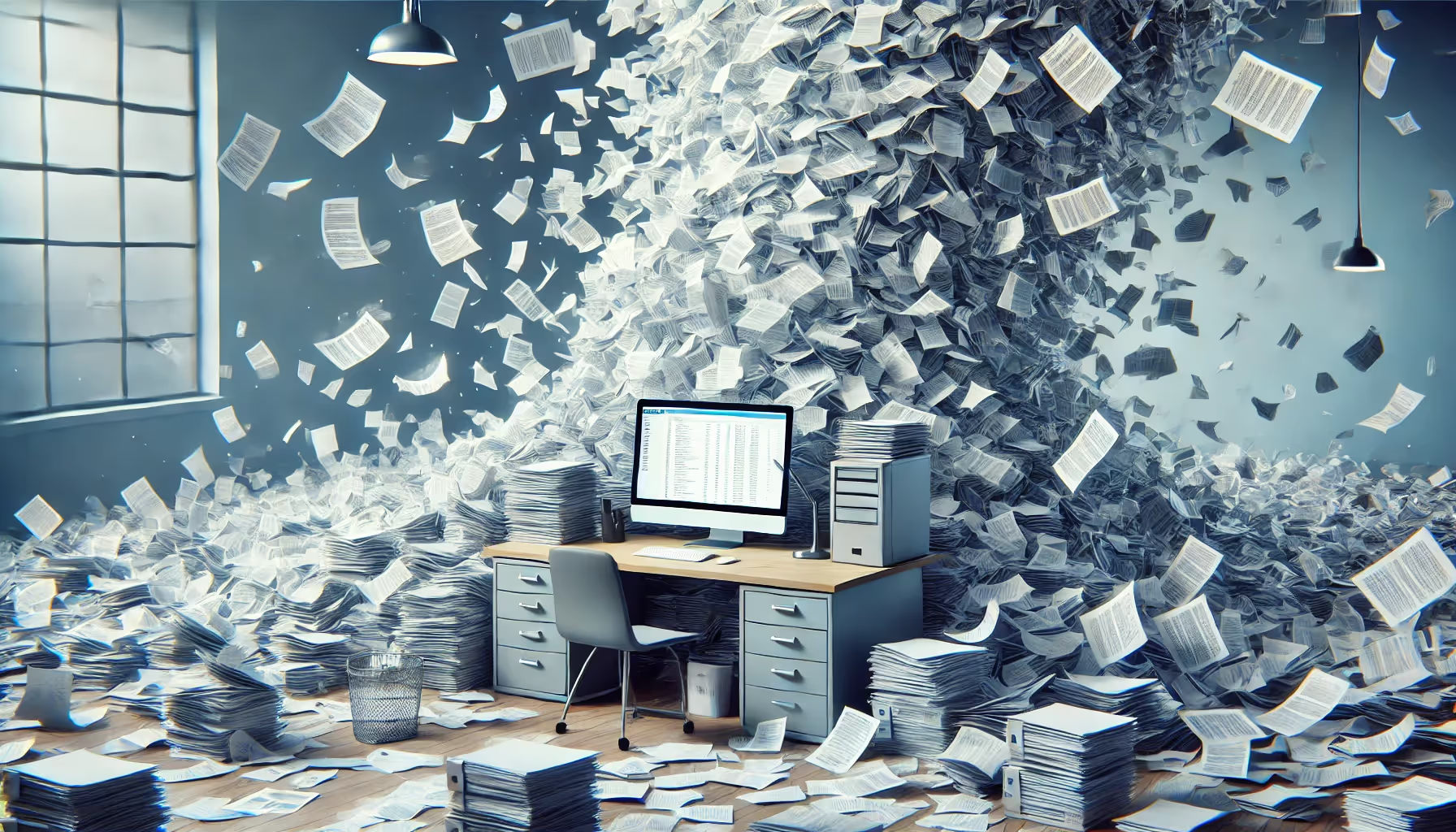
What Is AI-Generated Prior Art?
At a high-level, prior art may encompass any information (such as patent filings, webpages, scientific papers etc.) made available to the public prior to the priority/filing date of a patent application. Historically, this information was all produced by a human. This is changing with the rise of generative AI, because information is now being produced entirely devoid of human authorship. This shift has raised a few questions amongst patent practitioners and patent offices alike. For example, should AI-generated outputs qualify as prior art, in the same way as human disclosures? Should the conventional framework for assessing prior art be adapted? Indeed, the issue of AI-generated prior art may challenge traditional views on aspects such as relevance of prior art, whether a disclosure is enabling and accessible, or whether the volume of art affects a skilled person’s ability to use it.
Enablement and Enabling Disclosures in AI-Generated Prior Art
The principle of enablement means that, in order to be properly considered as prior art, a disclosure needs to provide enough detail for a skilled person to implement the subject matter of the disclosure in reality. Some AI generated content may pass this requirement, but some may not. For example, a prior art generator (such as allpriorart.com), that merely outputs a random amalgamation of features, may not provide an enabling disclosure, if it is unclear in the output how the features can be implemented together.
The European Patent Convention (EPC) requires prior disclosures to be both enabled and publicly accessible. Under EPC standards, the threshold for accessibility varies by disclosure type: patents require little corroboration for accessibility, while product sales demand more. As such, it may be possible, within the existing framework of European practice, to use this discretion to raise the bar for AI-generated disclosures, in terms of both accessibility and enablement.
The U.S. approach, which effectively presumes that cited prior art is enabling, may also require reconsideration. The current standard places the burden on applicants to prove that disclosures are non-enabling. This could become very burdensome when assessing vast quantities of speculative AI-generated content. Potentially, one could adjust this framework for AI-generated prior art, so that the burden only falls to the applicant in cases of prima facie anticipation in the AI-generated prior art of every feature of the invention at hand.
Accessibility of AI-Generated Prior Art
For a document to be prior art, it must be publically accessible. There is no need to prove that someone has actually looked at the document, but factors such as whether the document has been indexed and catalogued do play a part in determining the accessibility of the document.
Traditional prior art is generally documented and preserved in databases or academic repositories. Even webpages for example, are associated with metadata indicating authorship, edits, and a date of publication.
With respect to AI-generated prior art, the transient and unstructured nature of AI-generated information, and the sheer volume of it, raises concerns regarding public accessibility. If the volume of AI publications grows to a point where finding meaningful prior art becomes akin to finding a needle in a haystack, accessibility standards may need to be reevaluated specifically for AI-generated information.
On the other hand, AI is more and more frequently being used in search tools, optimising the capability of finding specific documents from a variety of sources. Improvements in search in this manner may somewhat negate the ‘needle in the haystack’ issue, meaning current accessibility standards may still yet be appropriate for AI-generated prior art. It will be interesting to see how this develops.
Policy Considerations
Recognizing the challenges AI-generated prior art poses, leading intellectual property organizations have already begun to question and address potential policy implications. A request for comments from the USPTO on this matter earlier this year elicited detailed responses from the Intellectual Property Owners Association (IPO) and the American Intellectual Property Law Association (AIPLA), for example. These responses reflect a need to develop a coherent framework for dealing with AI-generated information in a clear, fair, and repeatable manner.
As noted above, this could involve changes to the law governing prior art itself, changes to how existing law is implemented in practice, or merely acceptance of AI-generated prior art into existing practice standards with minimal change.
There are challenges with all three of these approaches. For example, there have been suggestions to change the law and/or practice standards in this area to effectively treat AI-generated information differently from information authored by a human. Of course, this would require one to firstly distinguish or classify prior art according to whether it is human or machine generated, which itself is not a simple task. Often AI-generated information can be unstructured, and very rarely a sufficient audit trail exists to be able to trace back information to its creation.
Conclusion
AI-generated prior art potentially poses complex, multifaceted challenges to the patent system, and may require a re-evaluation of how concepts such as novelty and nonobviousness are assessed. In view of this, patent authorities and stakeholders have already begun the first steps of shaping appropriate frameworks for the future.
Through thoughtful regulation, one might hope for a balanced framework that rewards inventors, while ensuring AI’s contributions to knowledge creation are recognized appropriately.
AI for patents.
Be 50%+ more productive. Join thousands of legal professionals around the World using Solve’s Patent Copilot™ for drafting, prosecution, invention harvesting, and more.